Pythonで画像認識してみたいです。
このような要望にお応えします。
今回は、YOLOv3の学習済みモデルを用いた物体検知をします。
下記のサイトを参考にさせていただきました。
- https://pjreddie.com/darknet/yolo/
- https://pjreddie.com/media/files/papers/YOLOv3.pdf
YOLOv3について
YOLOv3は、入力画像から物体の位置と種類を検出することができます。
YOLOv3の手法等の情報は、下記の論文を参照ください。
YOLOv3: An Incremental Improvement
- https://pjreddie.com/media/files/papers/YOLOv3.pdf
Google Colaboratoryの準備
・Googleのアカウントを作成します。
・Googleドライブにアクセスし、「新規」→「その他」から「Google Colaboratory」の順でクリックします。そうすると、Colaboratoryが起動します。
・Colaboratoryが起動したら、以下のコマンドをCoalboratoryのセルに入力し実行します。
そうすることで、Googleドライブをマウントします。
1 2 |
from google.colab import drive drive.mount('/content/drive') |
・実行後、認証コードの入力が促されます。このとき、「Go to this URL in a browser」が指しているURLにアクセスしgoogleアカウントを選択すると、認証コードが表示されますので、それをコピーしenterを押します。これでGoogleドライブのマウントが完了します。
YOLOv3の準備
YOLOv3の導入方法についての詳細情報は、下記を参照ください。
- https://pjreddie.com/darknet/yolo/
ツールをダウンロードする場所に移動します。本記事では、マイドライブにダウンロードします。
1 |
cd /content/drive/My Drive |
gitからツールをダウンロードします。
1 |
!git clone https://github.com/pjreddie/darknet |
ダウンロードしたフォルダに移動します。
1 |
cd darknet |
コンパイル/ビルドを実行します。
1 |
!make |
学習済みモデルのパラメータをダウンロードします。
1 |
!wget https://pjreddie.com/media/files/yolov3.weights |
サンプル画像を用いて、物体検知を実施してみます。
1 |
!./darknet detect cfg/yolov3.cfg yolov3.weights data/dog.jpg |
これで準備完了です。
以下のPythonスクリプトでdataフォルダに格納されたjpg画像に対して物体検知を行い、その結果をresultsフォルダに保存することができます。
1 2 3 4 5 6 7 8 9 10 11 |
import glob files = glob.glob('data/*.jpg') print(files) for index, file in enumerate(files): file = ' ' + file !./darknet detect cfg/yolov3.cfg yolov3.weights $file save_dir = ' ' + 'results/' + 'result' + str(index) !cp 'predictions.jpg' $save_dir |
出力結果
出力結果は、以下になります。
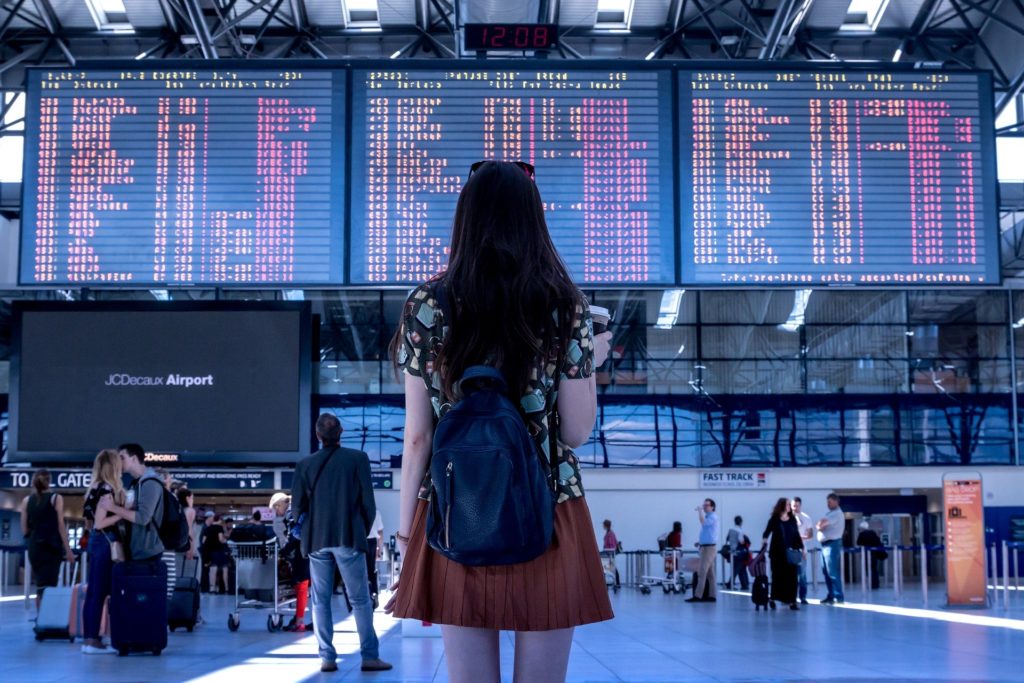
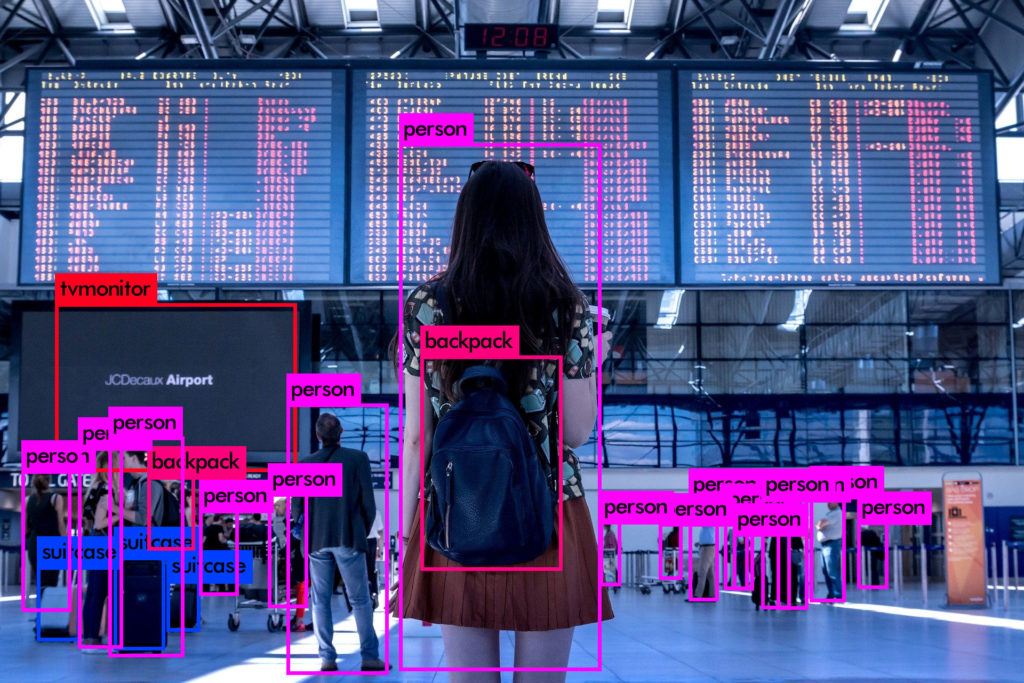
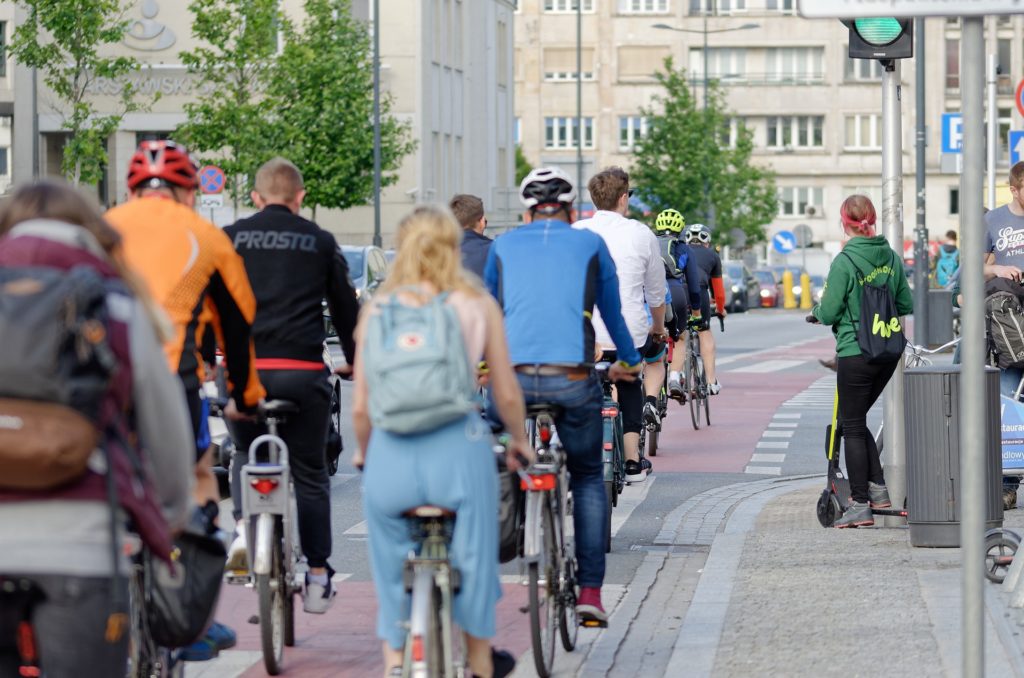
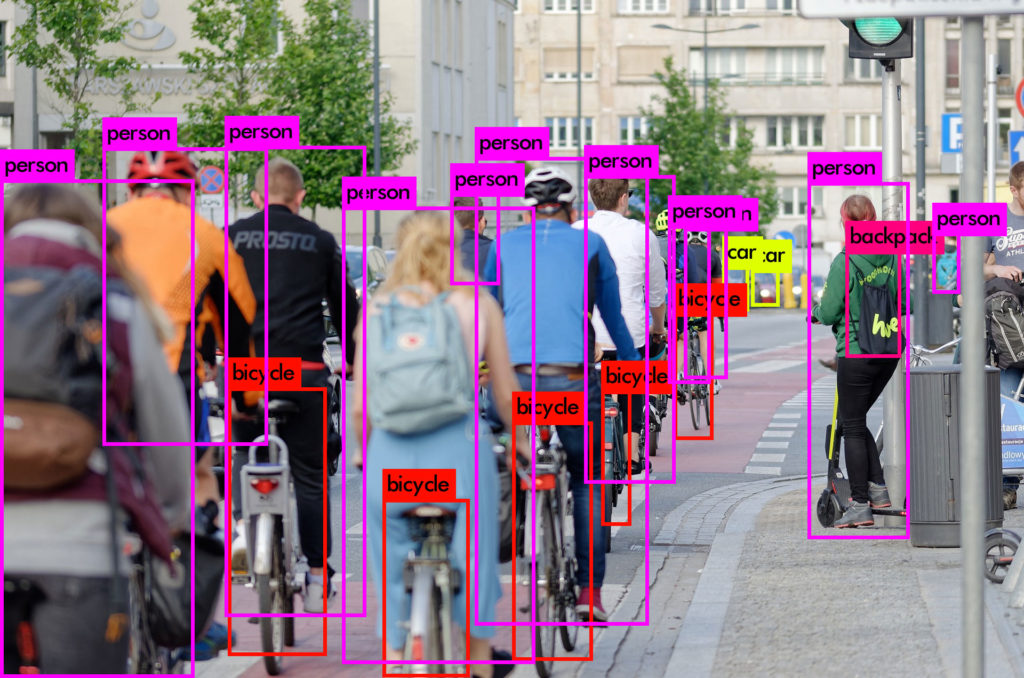
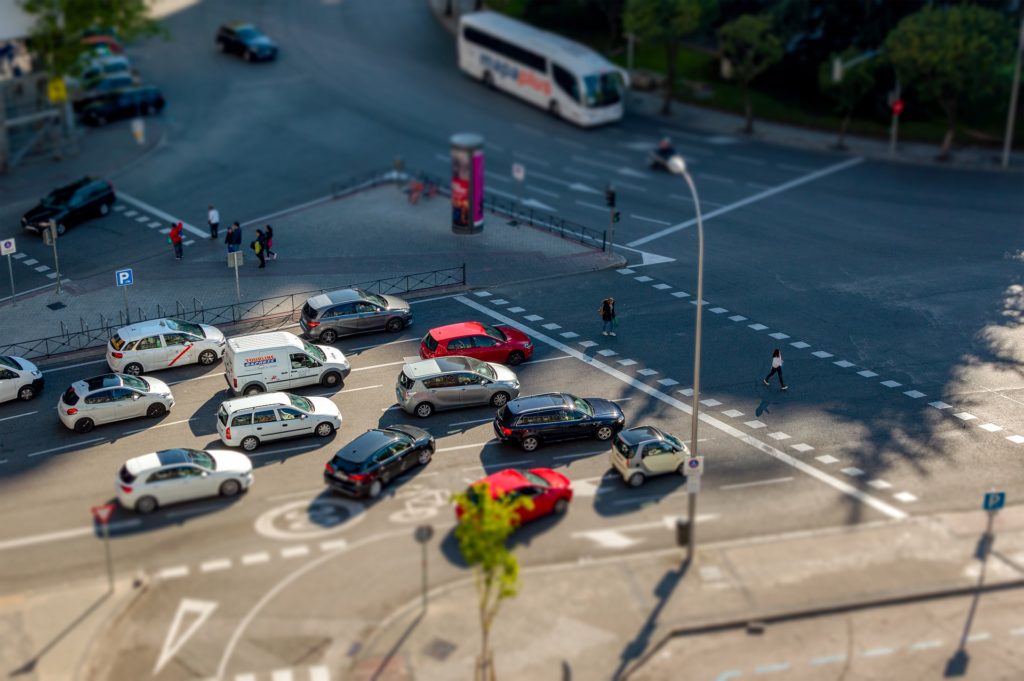
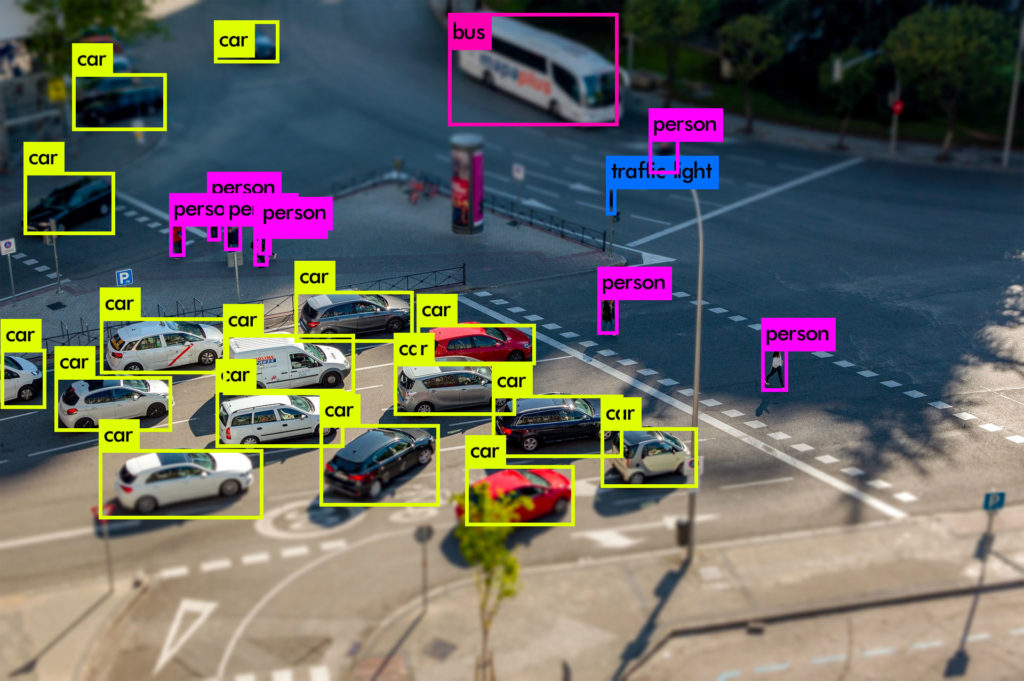
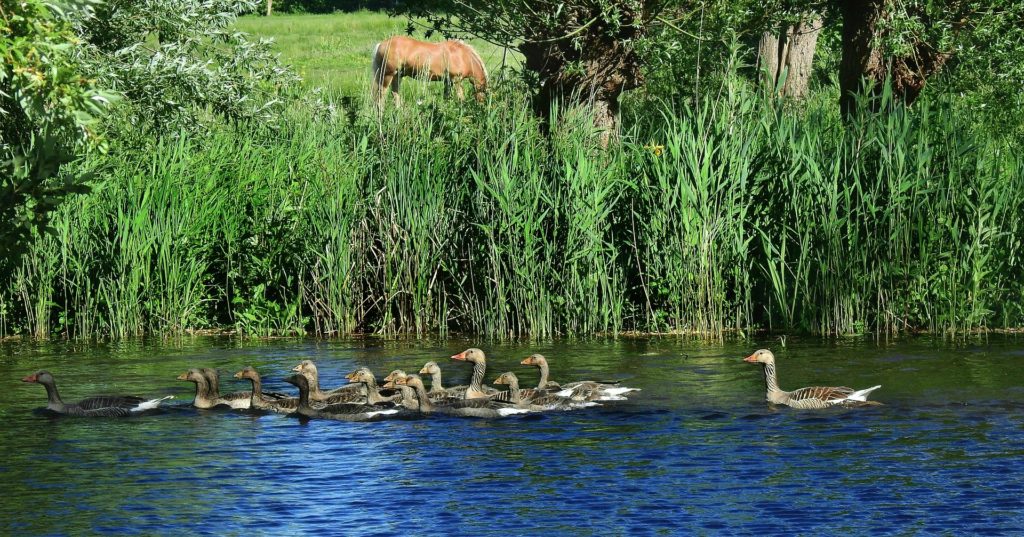
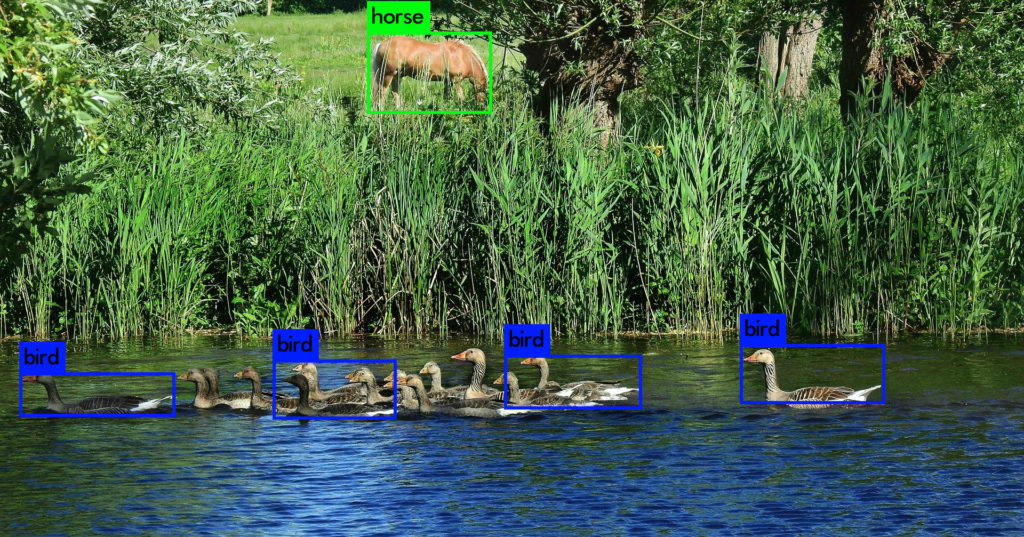
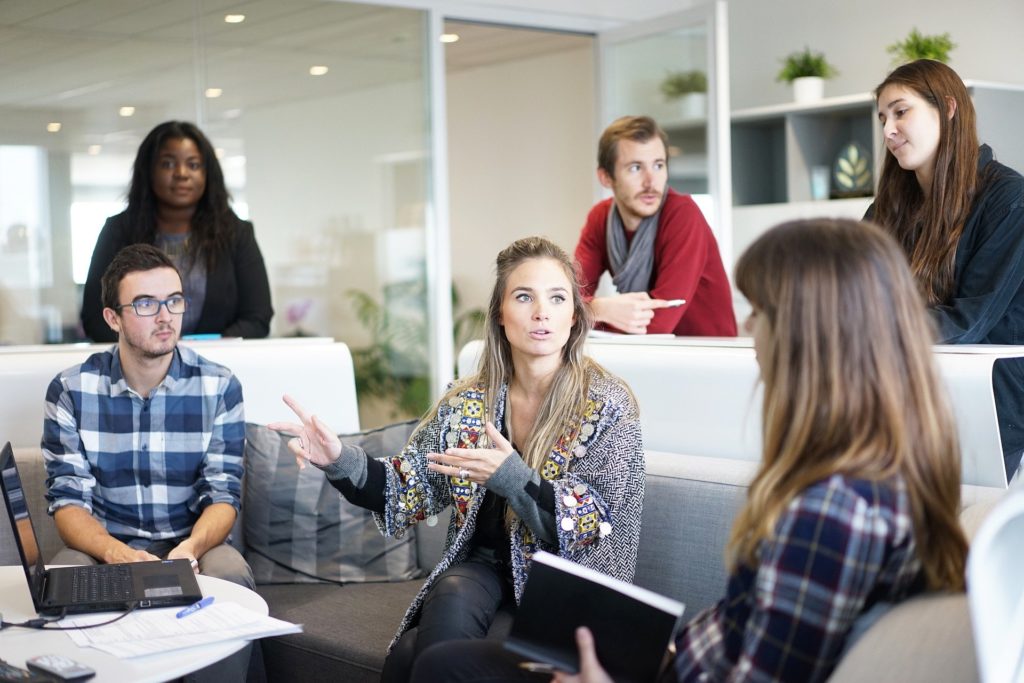
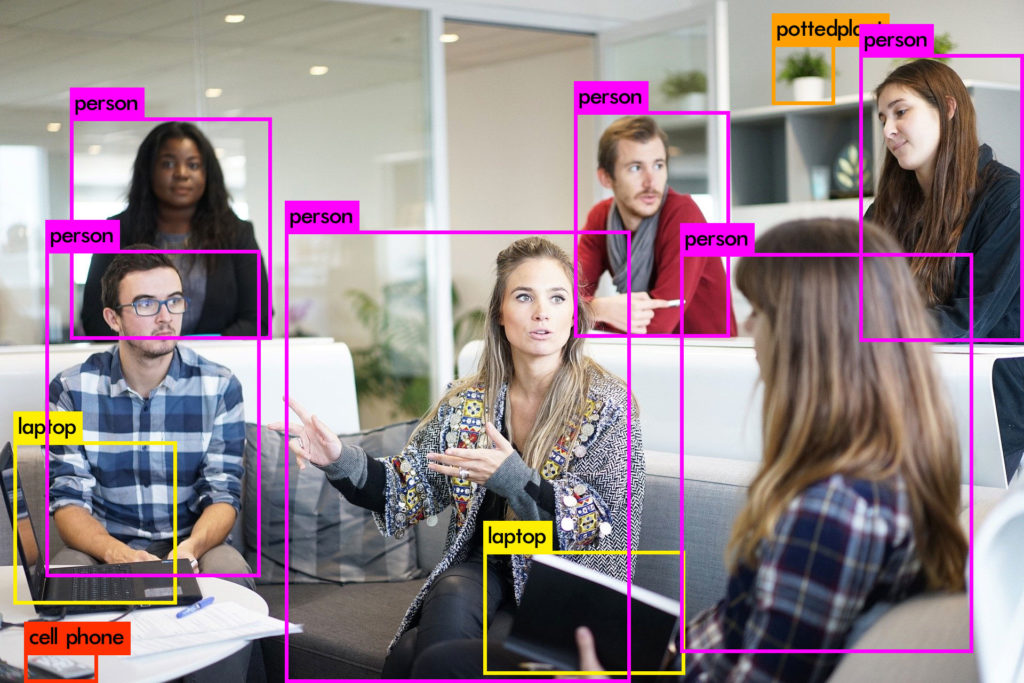
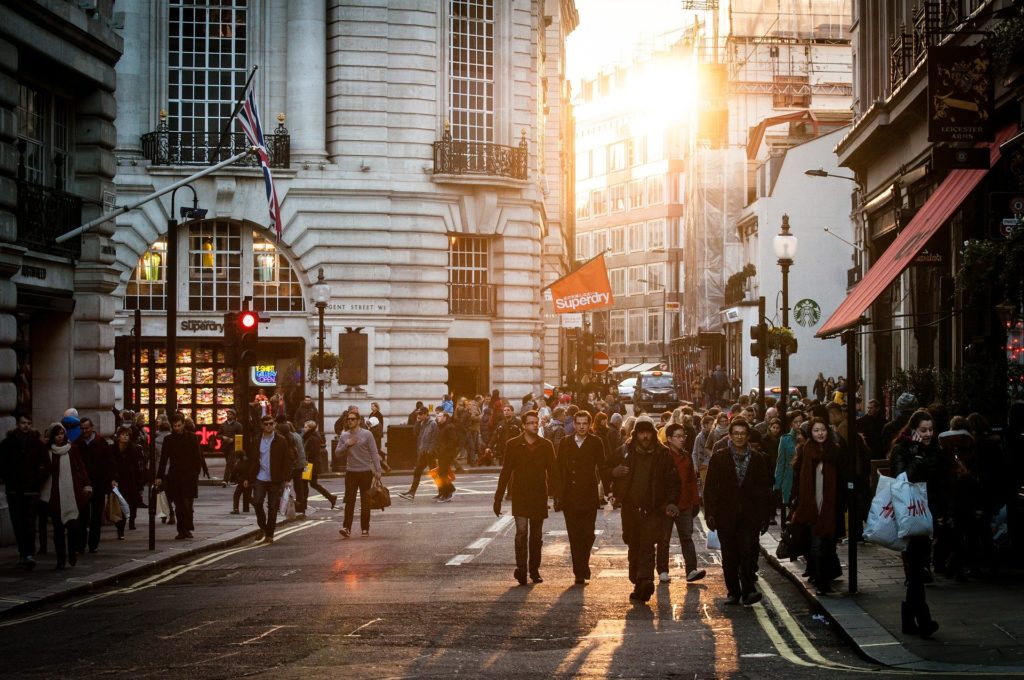
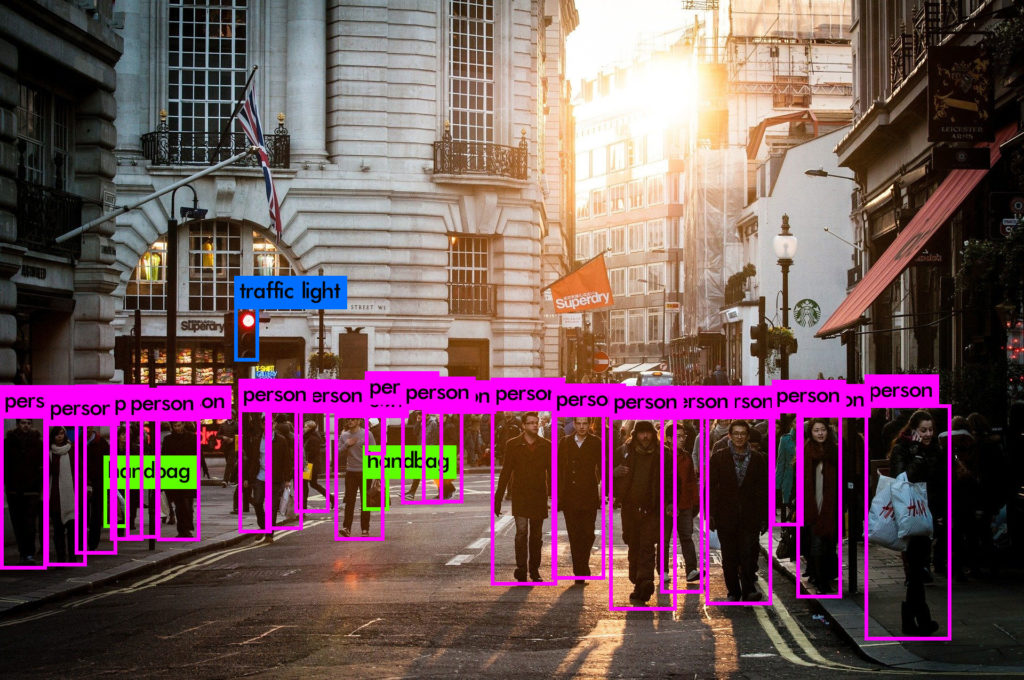
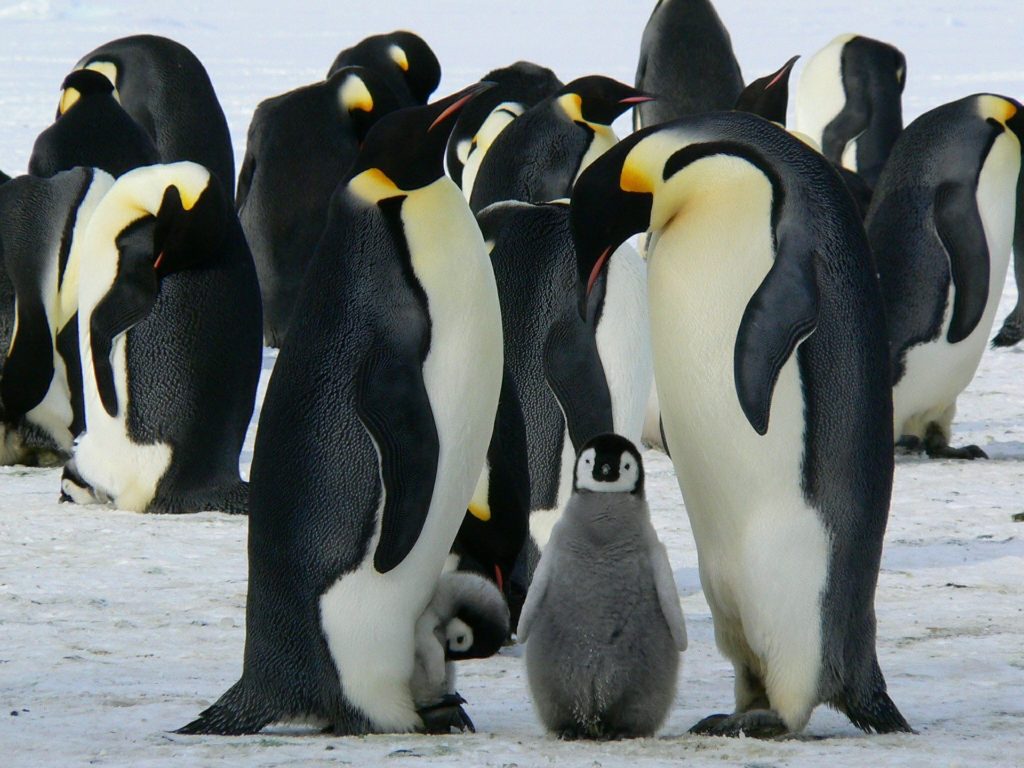
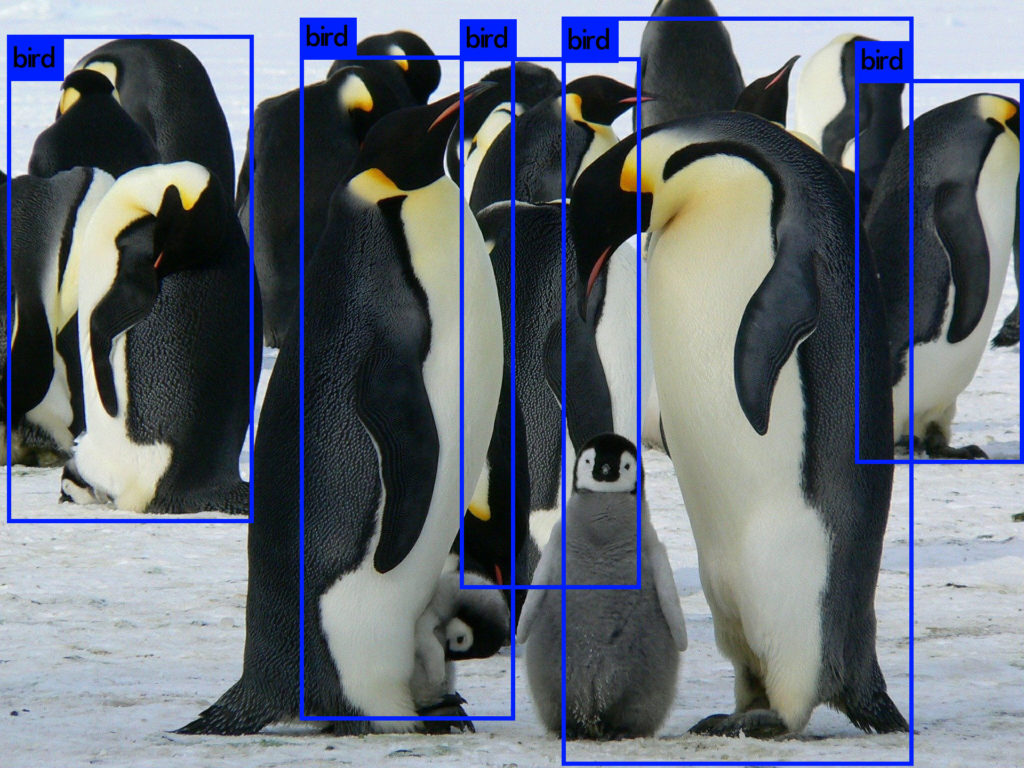
どうでしょうか?
様々な要素が混じる複雑な画像に対して実施してみるのもいいかもしれません。