Pythonで画像認識してみたいです。
このような要望にお応えします。
今回は、YOLOv4の学習済みモデルを用いた物体検知をします。
下記のサイトを参考にさせていただきました。
- https://github.com/AlexeyAB/darknet
- https://github.com/theAIGuysCode/YOLOv4-Cloud-Tutorial
- http://pjreddie.com/darknet/yolo/
- https://arxiv.org/abs/2004.10934
YOLOv4について
YOLOv4は、入力画像から物体の位置と種類を検出することができます。
YOLOv4の手法等の情報は、下記の論文を参照ください。
YOLOv4: Optimal Speed and Accuracy of Object Detection
- https://arxiv.org/abs/2004.10934
Google Colaboratoryの準備
・Googleのアカウントを作成します。
・Googleドライブにアクセスし、「新規」→「その他」から「Google Colaboratory」の順でクリックします。そうすると、Colaboratoryが起動します。
・Colaboratoryが起動したら、以下のコマンドをCoalboratoryのセルに入力し実行します。
そうすることで、Googleドライブをマウントします。
1 2 |
from google.colab import drive drive.mount('/content/drive') |
・実行後、認証コードの入力が促されます。このとき、「Go to this URL in a browser」が指しているURLにアクセスしgoogleアカウントを選択すると、認証コードが表示されますので、それをコピーしenterを押します。これでGoogleドライブのマウントが完了します。
YOLOv4の準備
YOLOv4の導入方法についてのより詳細な情報は、下記を参照ください。
- https://github.com/AlexeyAB/darknet
Google Colaboratoryの「ランタイム」→「ランタイムのタイプ変更」でGPUを選択します。
ツールをダウンロードする場所に移動します。本記事では、マイドライブにダウンロードします。
1 |
cd /content/drive/My Drive |
gitからツールをダウンロードします。
1 |
!git clone https://github.com/AlexeyAB/darknet |
ダウンロードしたフォルダに移動します。
1 |
cd darknet |
GPUとOpenCVを利用するようにmakeファイルを編集します。
1 2 3 4 |
!sed -i 's/OPENCV=0/OPENCV=1/' Makefile !sed -i 's/GPU=0/GPU=1/' Makefile !sed -i 's/CUDNN=0/CUDNN=1/' Makefile !sed -i 's/CUDNN_HALF=0/CUDNN_HALF=1/' Makefile |
コンパイル/ビルドを実行します。
1 |
!make |
YOLOv4学習済みのパラメータをダウンロードします。
1 |
!wget https://github.com/AlexeyAB/darknet/releases/download/darknet_yolo_v3_optimal/yolov4.weights |
下記のコマンドを実行することでdataフォルダ内のperson.jpgを読み込み、物体検知した結果がpredictions.jpgとして保存されます。
1 |
!./darknet detector test cfg/coco.data cfg/yolov4.cfg yolov4.weights data/person.jpg |
これで準備完了です。
以下のPythonスクリプトでdataフォルダに格納されたjpg画像に対して物体検知を行い、その結果をresultsフォルダに保存することができます。
1 2 3 4 5 6 7 8 9 10 11 |
import glob files = glob.glob('data/*.jpg') print(files) for index, file in enumerate(files): file = ' ' + file !./darknet detector test cfg/coco.data cfg/yolov4.cfg yolov4.weights $file save_dir = ' ' + 'results/' + 'result' + str(index) !cp 'predictions.jpg' $save_dir |
出力結果
出力結果は、以下になります。
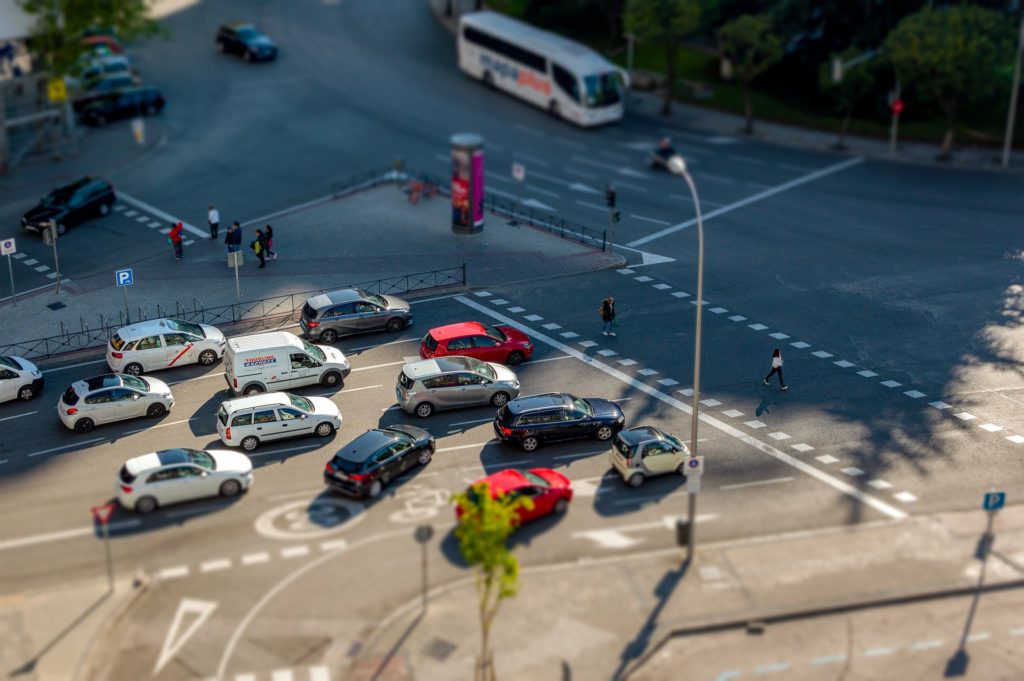
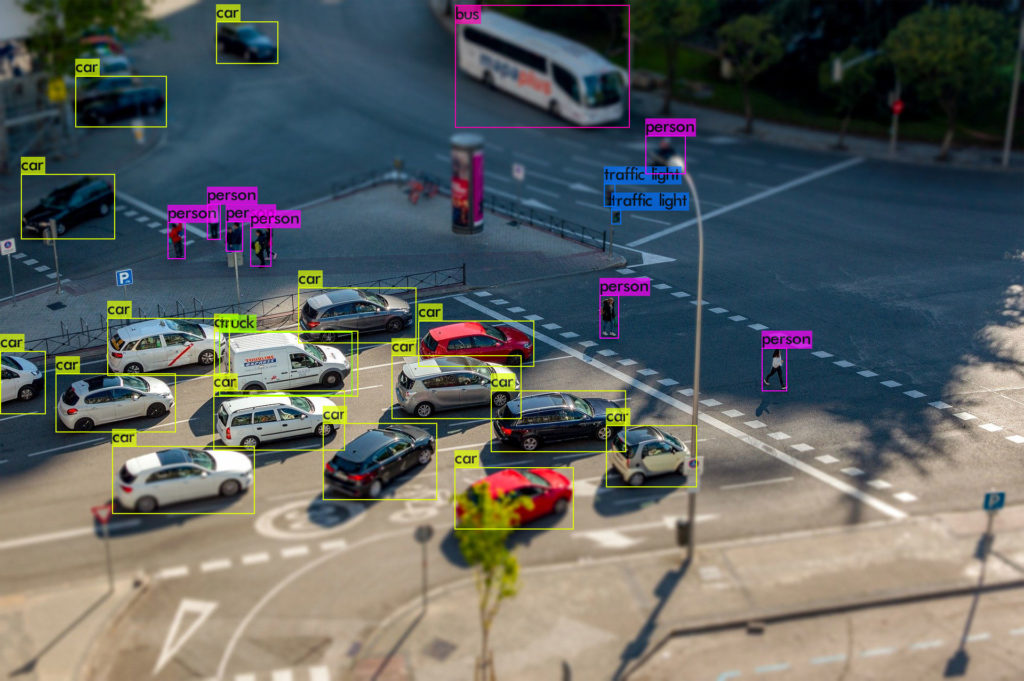
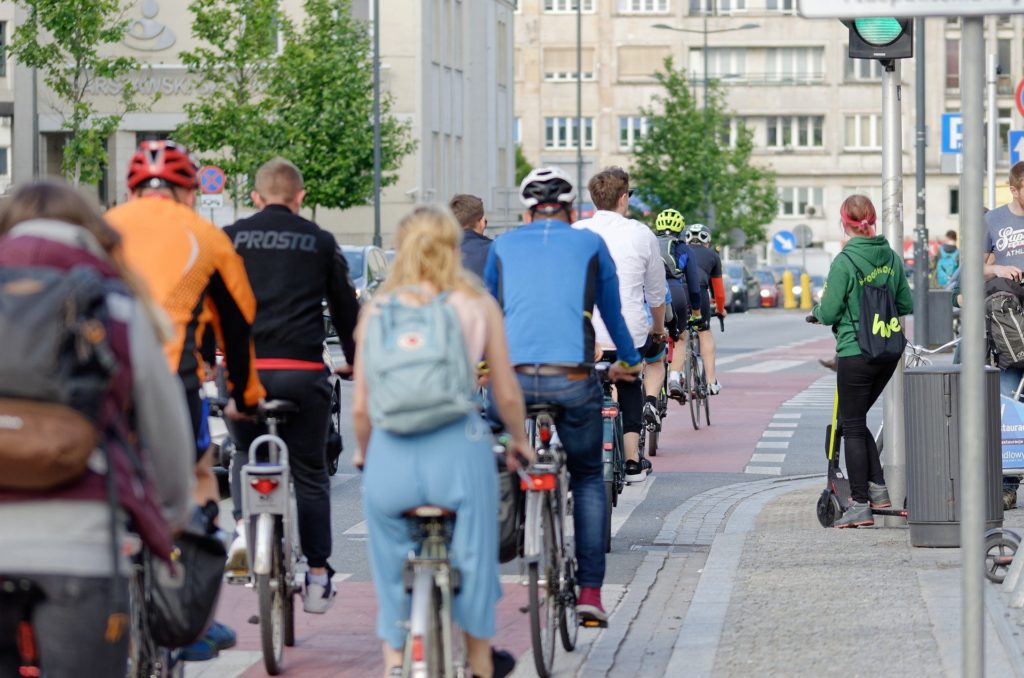
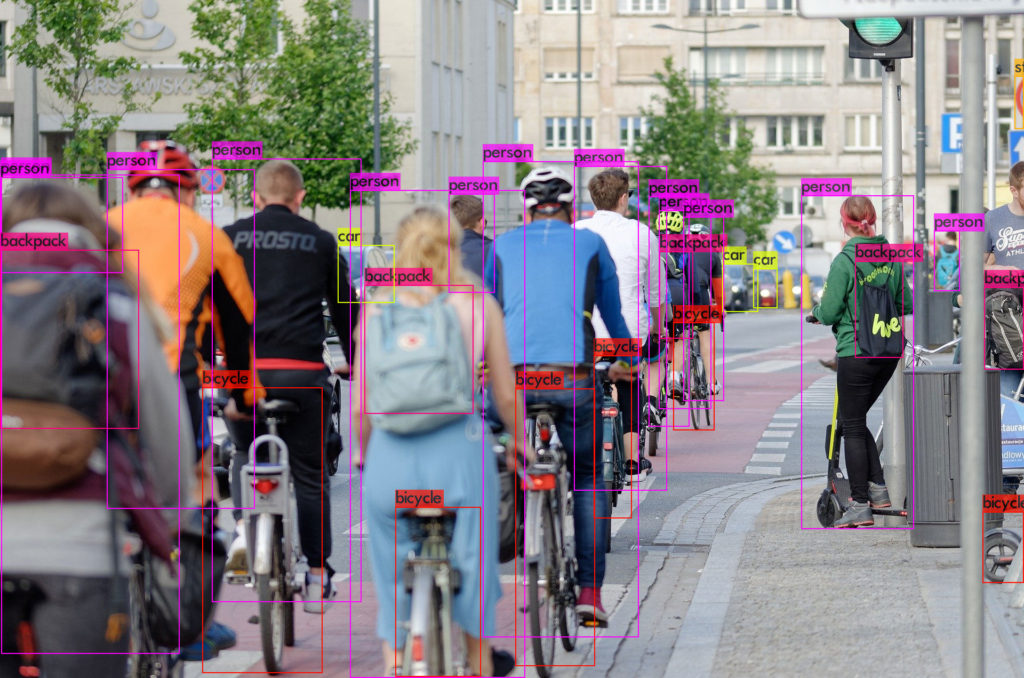
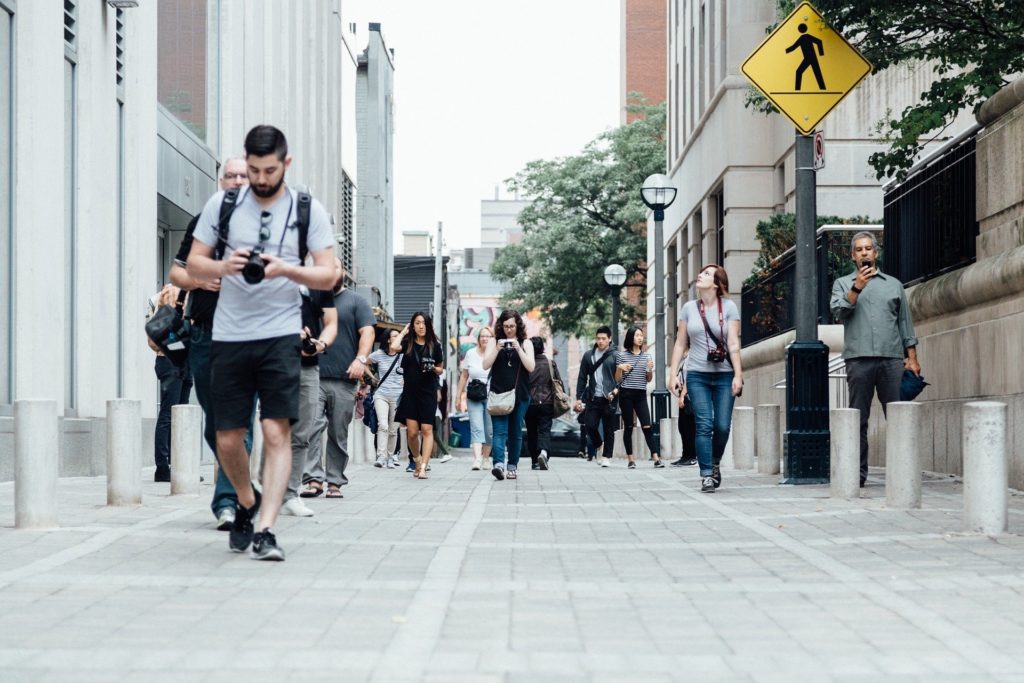
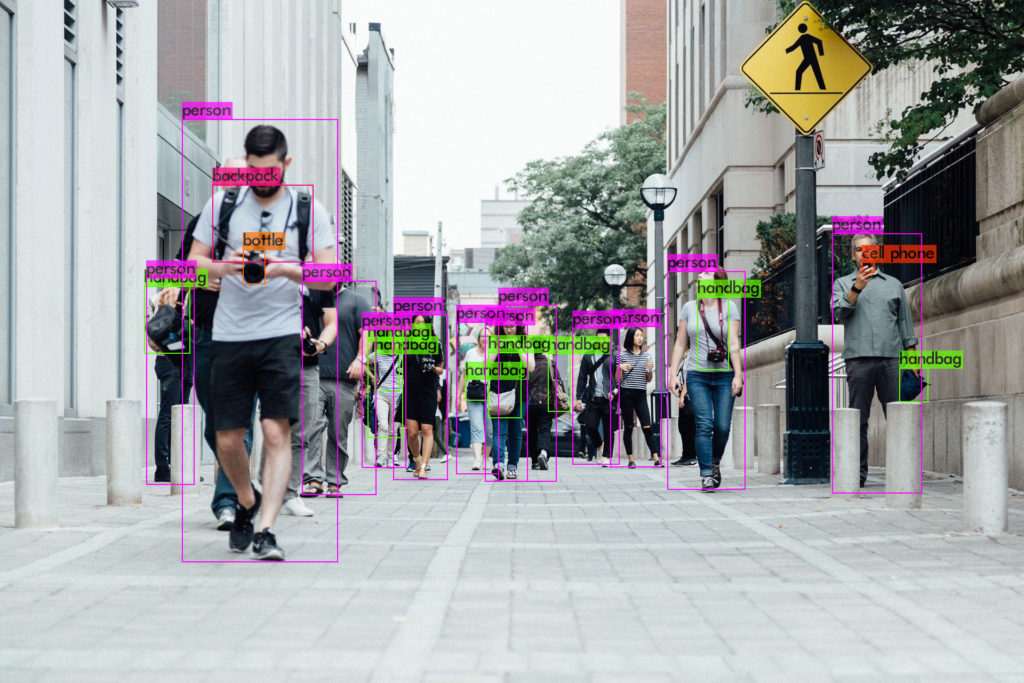
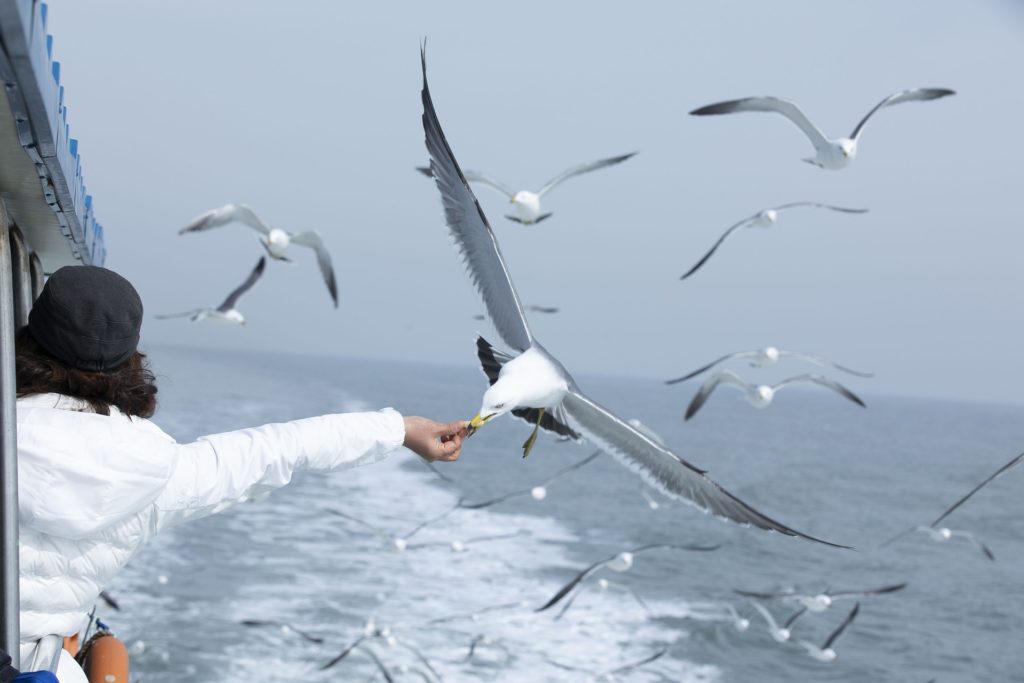
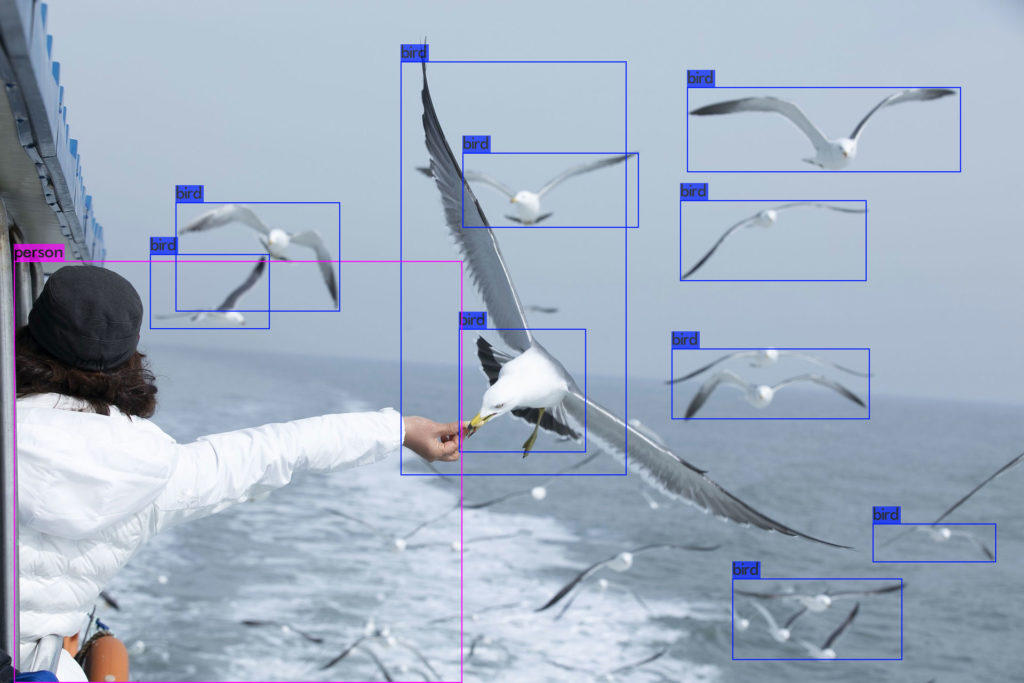
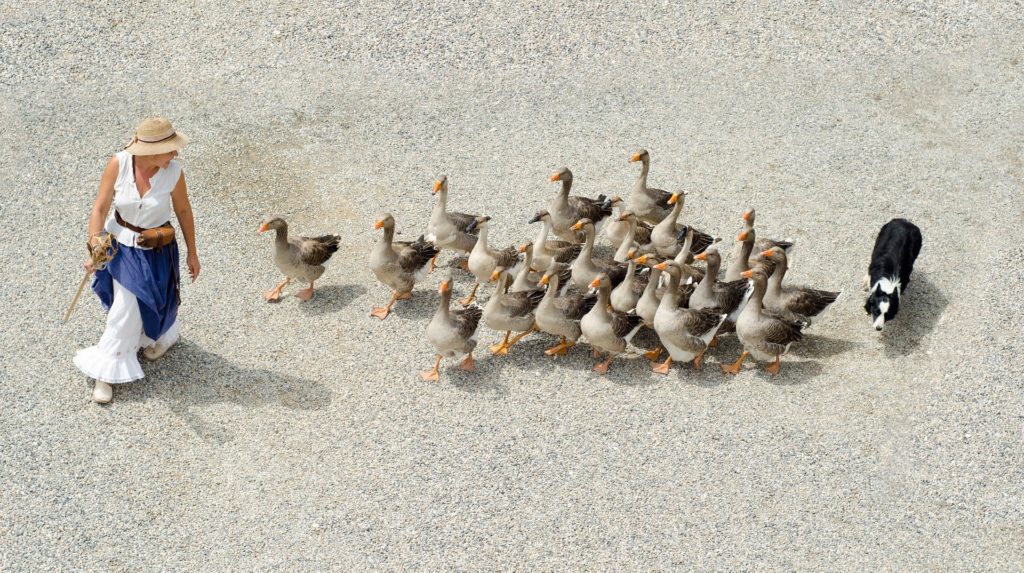
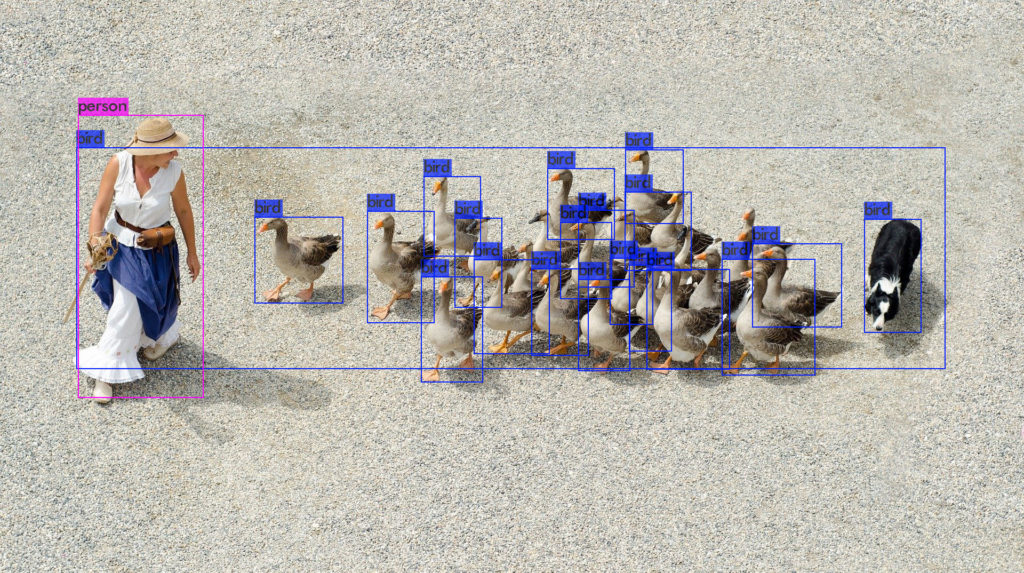
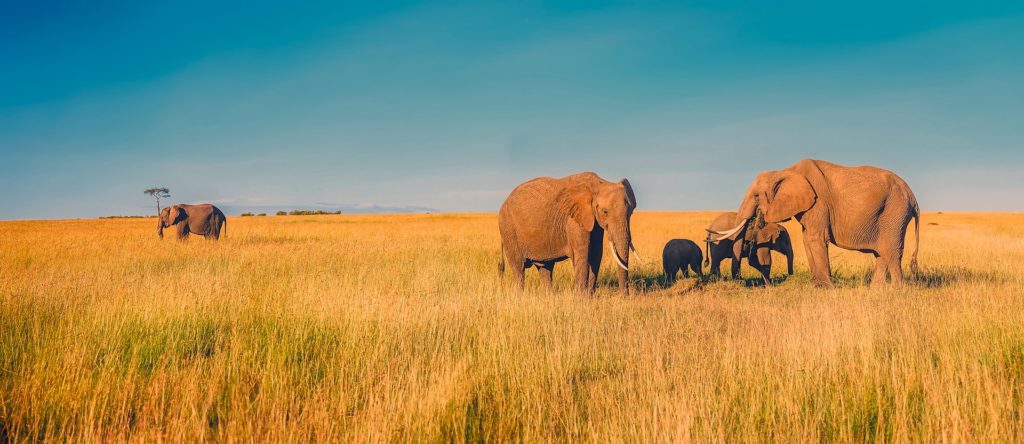
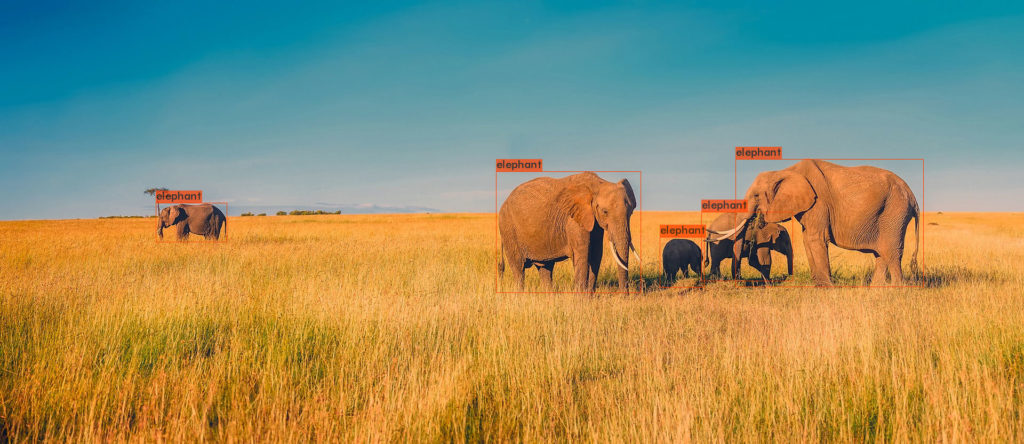
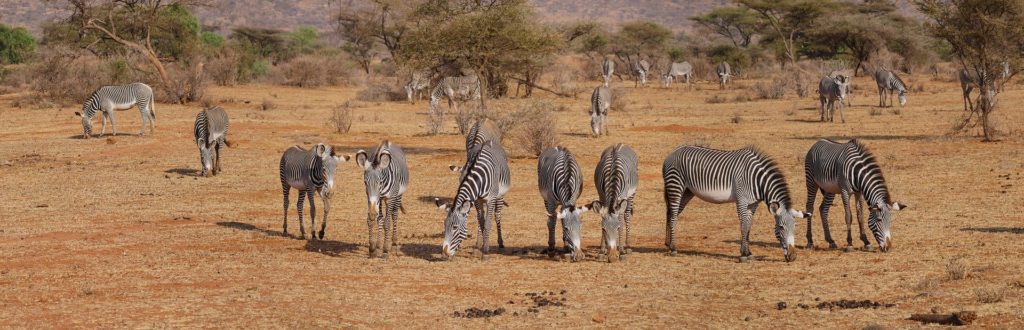
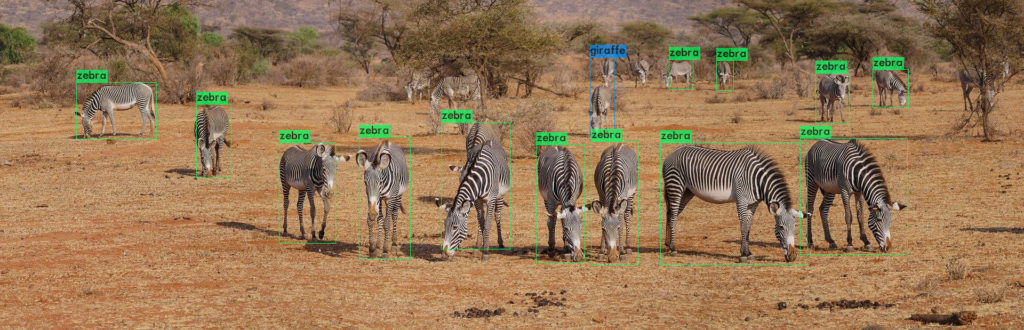
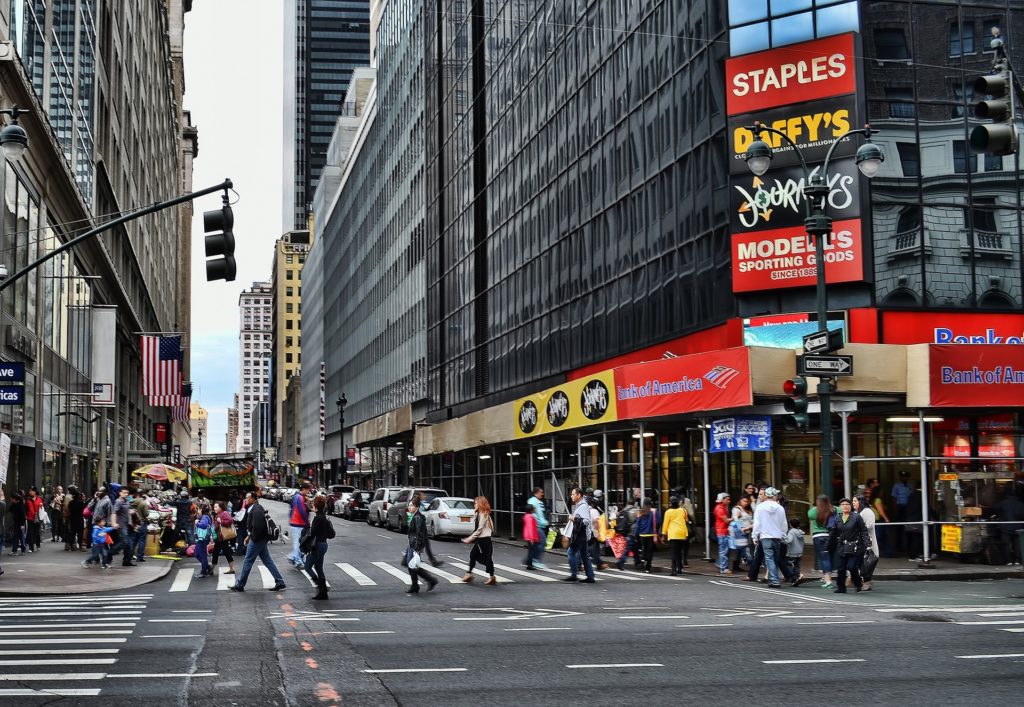
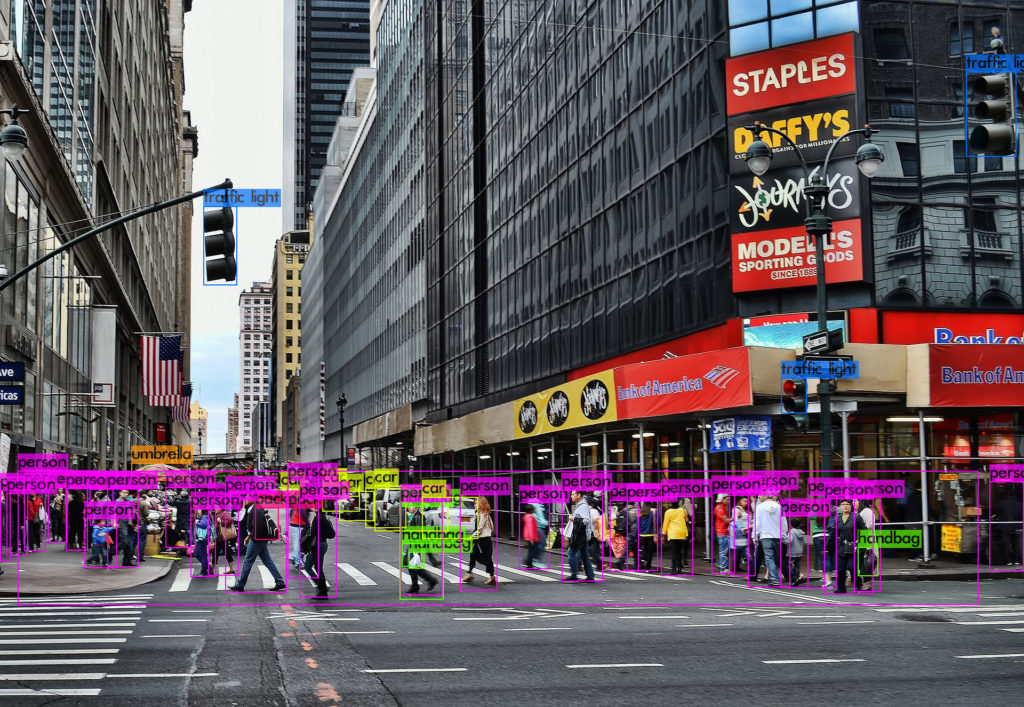
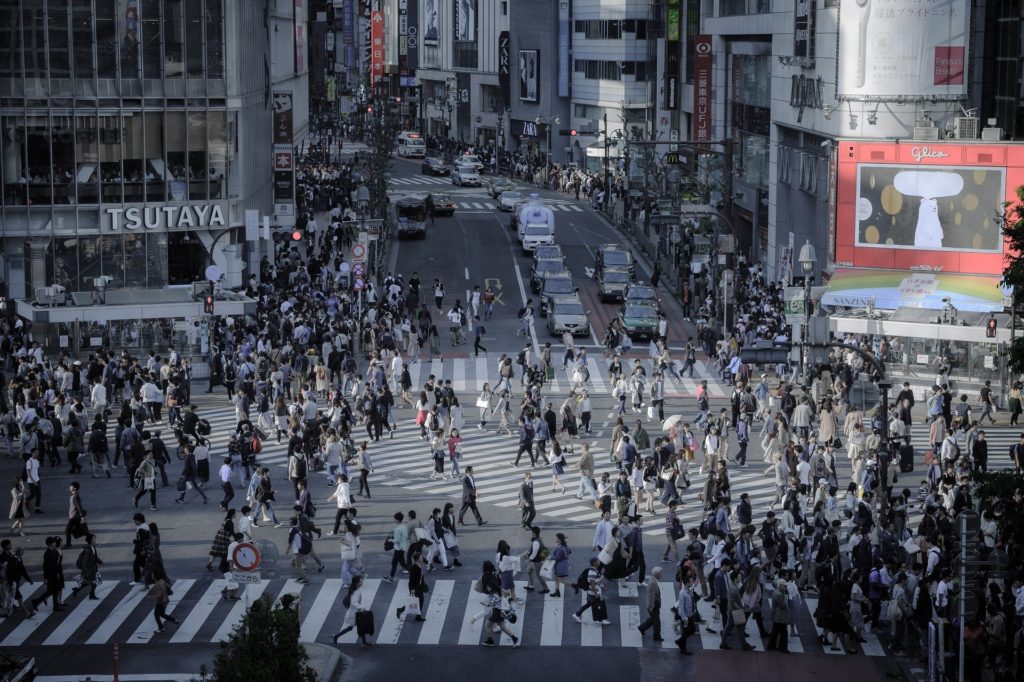
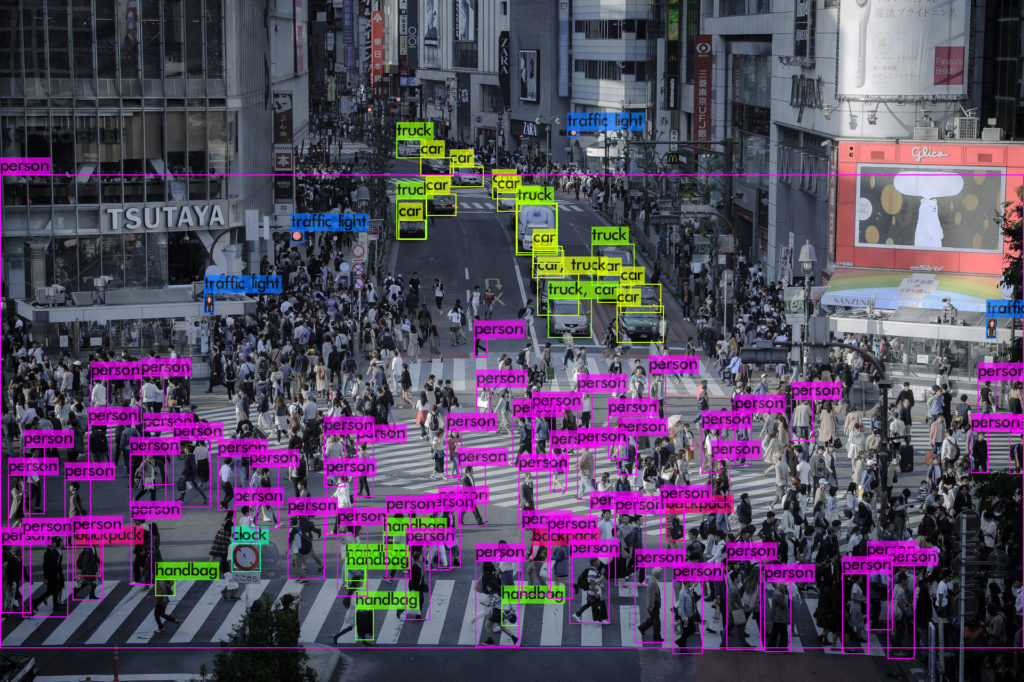
どうでしょうか? 様々な要素が混じる複雑な画像に対して実施してみるのもいいかもしれません。